This document is relevant for: Inf2
, Trn1
, Trn2
RMSNorm#
In this tutorial, we implement a kernel to perform RMSNorm of a 2D tensor, as described in Root Mean Square Layer Normalization. In doing so, we learn about:
The NKI syntax and programming model
Broadcasting tensors in different axis
Mapping embarrassingly parallel vector operations efficiently to the NeuronCore
Disable ineffectual data movement or compute within a tile using an execution mask
Before diving into RMSNorm of 2D input, let’s go over the RMSNorm operator for a
1D vector a
defined as below:
Note, g
is the RMSNorm weight, which has the same shape as the input vector a
.
The function RMS(a) produces a single scalar element, and we divide every element
in the input vector a
by the RMS(a)
scalar (i.e., a broadcast divide).
In Transformer models, we typically perform RMSNorm on a 2D input tensor instead
(with shape [sequence length, embedding size]
). 2D-RMSNorm simply performs 1D-RMSNorm
as discussed above for every row of the input 2D tensor. The g
RMSNorm weight vector
is shared (i.e., broadcasted) across the rows for the multiplication.
Figure below visualizes
the tensor shapes involved in 2D-RMSNorm, where a_tensor is the 2D input tensor and
g_tensor is the 1D RMSNorm weight:
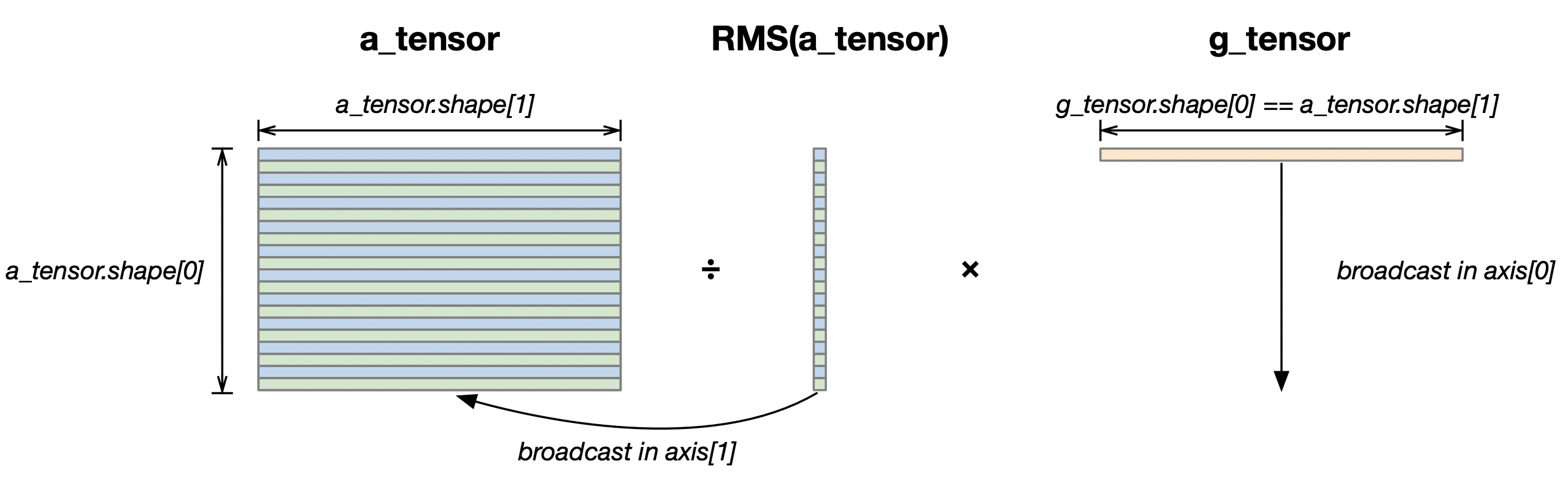
Fig. 85 RMSNorm tensor shapes#
We are going to map the rows (a_tensor.shape[0]
) to the partition dimension of the SBUF
once we load the tensor from HBM. This is a natural layout choice since each SBUF partition
has a one-to-one mapping to a parallel vector lane in the compute engines for calculating
RMS(a_tensor)
.
Note, the division of RMS(a_tensor)
requires broadcasting of one scalar across all elements
of a_tensor
within each partition, which is considered a free-axis broadcast and supported
by the flexible memory access pattern in hardware. On the other hand, the multiplication
with g_tensor requires broadcasting of a vector across all partitions, which is considered
a partition-axis broadcast and must invoke another instruction for the broadcasting
(broadcast_to()
API, details see below implementation) .
Compute kernel#
1import math
2import neuronxcc.nki as nki
3import neuronxcc.nki.language as nl
4
5
6@nki.jit
7def nki_rmsnorm_kernel(a_tensor, g_tensor):
8 # Calculate out_tensor = a_tensor/RMS(a_tensor) * g_tensor
9 # Where RMS(a_tensor) = sqrt((1/N) * sum(a_tensor * a_tensor))
10 # and N = a_tensor.shape[1]
11 # Reduction (mean) is performed in the free (2nd) dimension
12 out_tensor = nl.ndarray(a_tensor.shape, dtype=a_tensor.dtype,
13 buffer=nl.shared_hbm)
14
15 # Make sure shapes match
16 assert a_tensor.shape[1] == g_tensor.shape[0]
17
18 # Generate tensor indices to index input tensor
19 ix = nl.arange(128)[:, None]
20 iw = nl.arange(1)[:, None]
21 iy = nl.arange(a_tensor.shape[1])[None, :]
22
23 num_rows = a_tensor.shape[0]
24
25 # Load RMSNorm weight once, reused by rows/tiles of a_tensor
26 g_tile = nl.load(g_tensor.reshape((1, g_tensor.shape[0]))[iw, iy])
27
28 # Process 128 rows at a time due to 128-partition tile size limitation
29 # Since we're not reducing across the first dimension
30 # Tiles can be processed independently
31 for i in nl.affine_range(math.ceil(a_tensor.shape[0]/128)):
32
33 # Load input data from external memory to on-chip memory
34 a_tile = nl.load(a_tensor[i * 128 + ix, iy],
35 mask=(i * 128 + ix < num_rows))
36
37 # Compute element-wise square of a_tensor
38 in_square = nl.square(a_tile)
39
40 # Calculate sum of squared elements, along last dimension
41 square_sum = nl.sum(in_square, axis=[1])
42
43 # Scale and get a reciprocal
44 mean = square_sum / a_tensor.shape[1]
45
46 # Take square root of mean and then reciprocal with
47 # rsqrt API (one ISA instruction)
48 rms_reciprocal = nl.rsqrt(mean)
49
50 # Scale the input tensor
51 out_tile = nl.multiply(a_tile, rms_reciprocal)
52
53 # Broadcast weight along first axis to match tensor shape
54 # num_rows_active = min(num_rows - i * 128, 128)
55 g_bcast = g_tile.broadcast_to((128, g_tensor.shape[0]))
56
57 # Multiply with the RMSNorm weight
58 out_tile[...] = nl.multiply(out_tile, g_bcast,
59 mask=(i * 128 + ix < num_rows))
60
61 # store the addition results back to external memory (out_tensor)
62 nl.store(out_tensor[i * 128 + ix, iy], value=out_tile,
63 mask=(i * 128 + ix < num_rows))
64
65 return out_tensor
In this example, we implement RMSNorm for a 2D input tensor in nki_rmsnorm_kernel
:
We assume each SBUF partition is large enough to fit at least one row of
a_tensor
and one copy ofg_tensor
simultaneously.We load
g_tensor
once into the SBUF outside the main loop that iterates over tiles ofa_tensor
to achieve maximum reuse. The g_tensor is reshaped into a 2D tensor because SBUF is a two-dimensional memory and hence expects at least two dimension for any SBUF tensor. A reshape of an HBM tensor without changing the underlying storage format is in fact a no-op with no performance cost in the final compiled executable.To adhere to NKI’s tile-size considerations (Tile Size Considerations), we limit the partition axis size of
g_tensor
tile to be 128.The trip count of the compute loop is
math.ceil(a_tensor.shape[0]/128)
. In cases wherea_tensor.shape[0]
is not a multiple of 128, we can disable ineffectual data movement or compute in the last iteration using themask
field (discussions below).Within the compute loop:
We load one tile of
g_tensor
with shape(128, g_tensor.shape[1])
usingnl.load
API. We guard the loading boundary by specifyingmask=(i * 128 + ix < num_rows)
, which ensures we don’t access out-of-bound memory when the number of rows ina_tensor
is not a multiple of 128.We perform the free-axis broadcast multiply (
division of RMS(a)
) usingnl.multiply(a_tile, rms_reciprocal)
, which is lowered into nki.isa.tensor_scalar instruction under the hood.To broadcast multiply with the RMSNorm weight g_tensor, we need to perform a partition-axis broadcast of the g_tensor. The number of partitions to broadcast to depends on how many active rows are being normalized in the current loop iteration:
min(num_rows - i * 128, 128)
. Next, we can do element-wise multiplication of the broadcastedg_tensor
and the intermediate normalized tileout_tile
, which is lowered into nki.isa.tensor_tensor instruction under the hood.Finally, we store the normalized tile back into HBM using the nl.store API. We guard the store boundary similar to load boundary using the
mask
field.
Launching kernel and testing correctness#
PyTorch#
Below we write a reference PyTorch implementation of RMSNorm and verify our NKI kernel output against the reference in the same script as the kernel.
1# Reference torch implementation
2def torch_rmsnorm_kernel(a_tensor, g_tensor):
3 # Square the tensor (element-wise)
4 in_square = a_tensor.pow(2)
5 # Calculate means in the free dimension
6 mean = in_square.mean(dim=1, keepdim=True)
7 # Scale by reciprocal of sqrt(mean)
8 tensor = a_tensor * torch.rsqrt(mean)
9
10 # Scale the output by the weight
11 return tensor * g_tensor
12
13from torch_xla.core import xla_model as xm
14device = xm.xla_device()
15
16a_tensor = torch.rand((250, 512), dtype=torch.float32).to(device=device)
17g_tensor = torch.rand((512), dtype=torch.float32).to(device=device)
18
19output_nki = nki_rmsnorm_kernel(a_tensor, g_tensor)
20print(f"output_nki={output_nki}")
21
22output_torch = torch_rmsnorm_kernel(a_tensor, g_tensor)
23print(f"output_torch={output_torch}")
24
25if torch.allclose(output_torch, output_nki, atol=1e-5, rtol=1e-3):
26 print("NKI and Torch match")
27else:
28 print("NKI and Torch differ")
Output:
2024-07-27 15:22:50.000670: 7592 INFO ||NEURON_CACHE||: Compile cache path: /var/tmp/neuron-compile-cache
2024-07-27 15:22:50.000672: 7592 INFO ||NEURON_CC_WRAPPER||: Call compiler with cmd: neuronx-cc compile --target=trn1 --framework=XLA /tmp/ubuntu/neuroncc_compile_workdir/54c8e689-108c-433e-832a-f9282acdf114/model.MODULE_7170924315921358669+d41d8cd9.hlo_module.pb --output /tmp/ubuntu/neuroncc_compile_workdir/54c8e689-108c-433e-832a-f9282acdf114/model.MODULE_7170924315921358669+d41d8cd9.neff --verbose=35
DGE ON Levels: {'scalar_dynamic_offset', 'io'}
.
Compiler status PASS
output_nki=tensor([[0.8418, 1.3092, 0.7372, ..., 0.1458, 0.8831, 0.2339],
[0.1745, 0.3416, 0.1519, ..., 0.3358, 0.1832, 0.4795],
[0.0111, 1.1799, 0.8628, ..., 0.3107, 0.8328, 0.5663],
...,
[1.1213, 0.5449, 0.3020, ..., 0.4050, 0.4838, 0.0834],
[0.8246, 0.5027, 0.2745, ..., 0.4069, 1.0456, 1.0978],
[0.6415, 0.3637, 0.1462, ..., 0.2441, 1.0535, 0.4138]],
device='xla:0')
2024-07-27 15:22:51.000907: 7592 INFO ||NEURON_CACHE||: Compile cache path: /var/tmp/neuron-compile-cache
2024-07-27 15:22:51.000908: 7592 INFO ||NEURON_CC_WRAPPER||: Call compiler with cmd: neuronx-cc compile --target=trn1 --framework=XLA /tmp/ubuntu/neuroncc_compile_workdir/6d2046fc-c02d-4d3d-8746-50399ad50832/model.MODULE_18272098496972694952+d41d8cd9.hlo_module.pb --output /tmp/ubuntu/neuroncc_compile_workdir/6d2046fc-c02d-4d3d-8746-50399ad50832/model.MODULE_18272098496972694952+d41d8cd9.neff --verbose=35
DGE ON Levels: {'scalar_dynamic_offset', 'io'}
.
Compiler status PASS
output_torch=tensor([[0.8418, 1.3092, 0.7372, ..., 0.1458, 0.8831, 0.2339],
[0.1745, 0.3416, 0.1519, ..., 0.3358, 0.1832, 0.4795],
[0.0111, 1.1799, 0.8628, ..., 0.3107, 0.8328, 0.5663],
...,
[1.1213, 0.5449, 0.3020, ..., 0.4050, 0.4838, 0.0834],
[0.8246, 0.5027, 0.2745, ..., 0.4069, 1.0456, 1.0978],
[0.6415, 0.3637, 0.1462, ..., 0.2441, 1.0535, 0.4138]],
device='xla:0')
2024-07-27 15:22:53.000466: 7592 INFO ||NEURON_CACHE||: Compile cache path: /var/tmp/neuron-compile-cache
2024-07-27 15:22:53.000467: 7592 INFO ||NEURON_CC_WRAPPER||: Call compiler with cmd: neuronx-cc compile --target=trn1 --framework=XLA /tmp/ubuntu/neuroncc_compile_workdir/32c983cd-2c40-4723-8342-d4422107708c/model.MODULE_968738949480579147+d41d8cd9.hlo_module.pb --output /tmp/ubuntu/neuroncc_compile_workdir/32c983cd-2c40-4723-8342-d4422107708c/model.MODULE_968738949480579147+d41d8cd9.neff --verbose=35
DGE ON Levels: {'io', 'scalar_dynamic_offset'}
.
Compiler status PASS
NKI and Torch match
JAX#
Below we write a reference JAX implementation of RMSNorm and verify our NKI kernel output against the reference in the same script as the kernel.
1# Reference JAX implementation
2def jax_rms_norm(a_tensor, g_tensor):
3 # Square the tensor (element-wise)
4 in_square = jnp.square(a_tensor)
5 # Calculate means in the free dimension
6 mean = in_square.mean(axis=1, keepdims=True)
7 # Scale by reciprocal of sqrt(mean)
8 tensor = a_tensor * jnp.reciprocal(jnp.sqrt(mean))
9
10 # Scale the output by the weight
11 return tensor * g_tensor
12
13a_key, g_key = jax.random.split(jax.random.PRNGKey(42))
14a_tensor = jax.random.uniform(a_key, (250, 512))
15g_tensor = jax.random.uniform(g_key, (512,))
16
17output_nki = nki_rmsnorm_kernel(a_tensor, g_tensor)
18
19print(a_tensor)
20
21print(f"output_nki={output_nki}")
22
23output_jax = jax_rms_norm(a_tensor, g_tensor)
24print(f"output_jax={output_jax}")
25
26if jnp.allclose(output_jax, output_nki, atol=1e-5, rtol=1e-3):
27 print("NKI and JAX match")
28else:
29 print("NKI and JAX differ")
Download All Source Code#
Click the links to download source code of the kernels and the testing code discussed in this tutorial.
NKI baremetal implementation:
rmsnorm_nki_kernels.py
PyTorch reference implementation:
rmsnorm_torch.py
JAX reference implementation:
rmsnorm_jax.py
You can also view the source code in the GitHub repository nki_samples
Example usage of the scripts:#
Run NKI baremetal implementation:
python3 rmsnorm_nki_kernels.pyRun PyTorch implementation:
python3 rmsnorm_torch.pyRun JAX implementation:
python3 rmsnorm_jax.py
This document is relevant for: Inf2
, Trn1
, Trn2